Tropical cyclone study from remote sensing data with machine learning approach.
Introduction
In INSTeG, one of the active research is studying tropical cyclone parameters from remote sensing satellite including scatterometer and altimeter using machine learning approach (e.g. Artificial Neural Network (ANN), Support Vector Machine (SVM), Gaussian Process Regression (GPR) and Random Forest). In complex and extreme environment such as tropical cyclone, algorithm developed in neutral atmospheric condition become an ill-posed solution. Thus, reducing the accuracy of the estimates variable. With the unique advantage and intelligent computational capability, lots of satellite observed parameters can be exploit to derive more accurate tropical cyclone variables.
Active Microwave Satellite for Tropical Cyclone (TC) Study
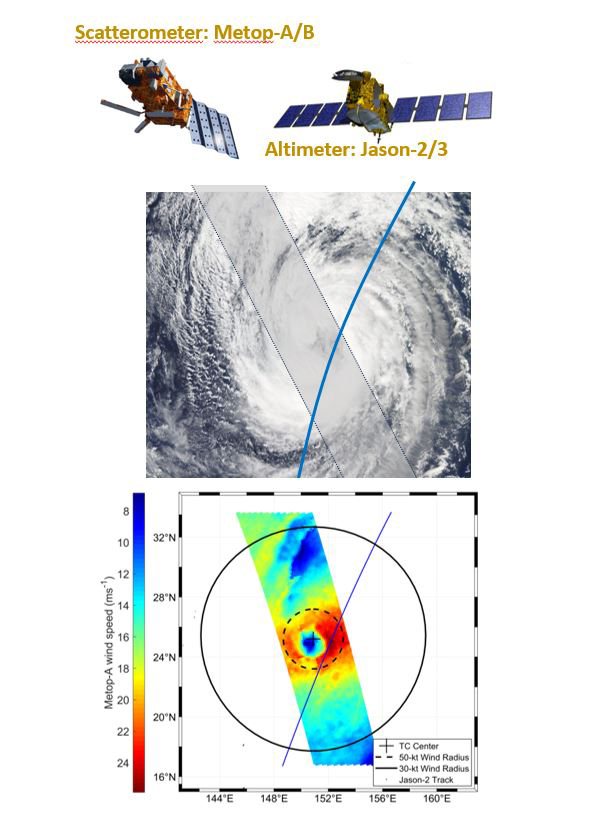
Altimeter and scatterometer satellite crossing Typhoon Choi-Wan with their corresponding tracks and measured data. Polar orbiting scatterometer and altimeter satellite can provide global and consistent observation of tropical cyclone. Multiple sensors measuring simultaneous parameters such as backscatter, waves, brightness temperature, atmospheric water content, and wind speed can be explore further to understand the physical dynamic of tropical cyclone.
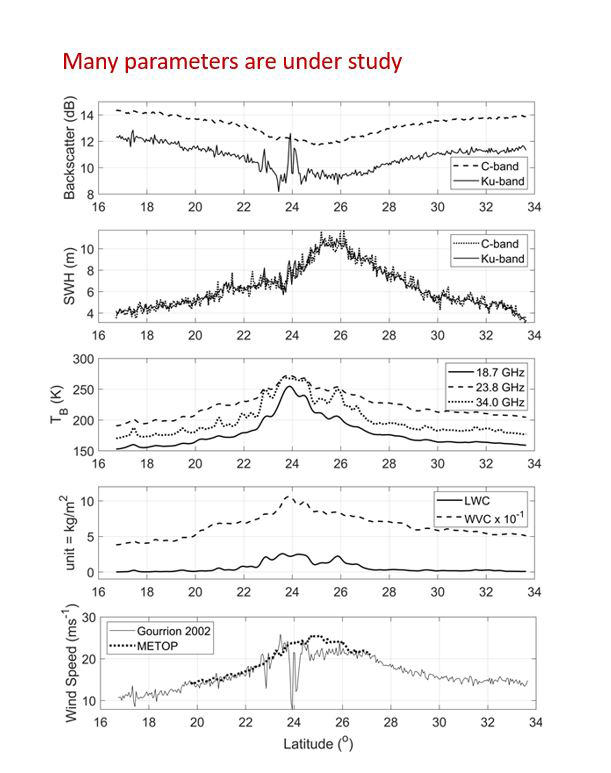
Overlay of different parameters observed by Altimeter as it crossing Typhoon Choi-Wan. Ocean-Atmospheric parameters are displayed and critically analyses to investigate their physical inter-relations.
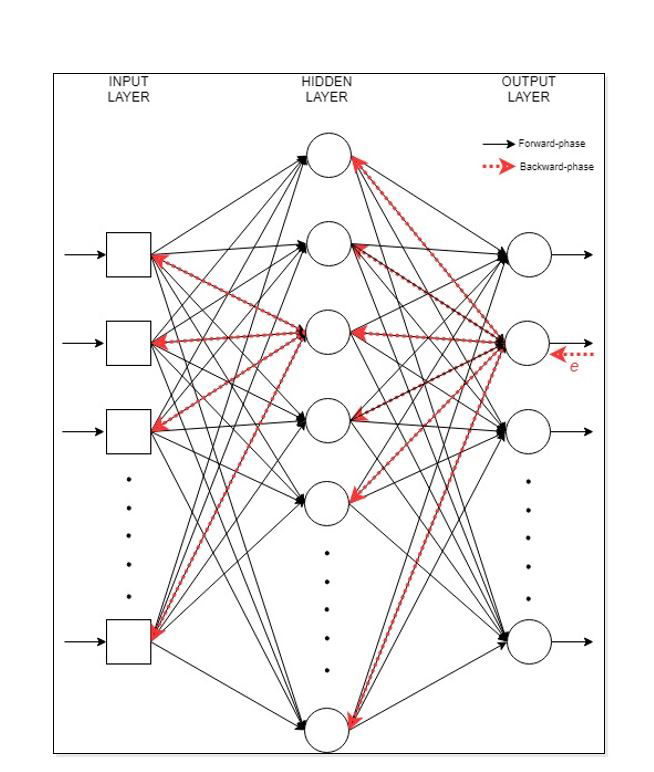
ANN model framework in which multiple parameters are exploits to derived more accurate parameters (e.g.: wind speed)
The complex multi-dimensional relation exists inside extreme environment can be resolve by using machine learning.

More accurate parameters can be extracted from the machine learning output such as the Maximum Sustained Wind, the Radius of Gale-force (R-30kt), and the Radius of Hurricane-force (R-50kt).This can provide more accurate information to estimate the tropical cyclone size, intensity and strength for emergency action.